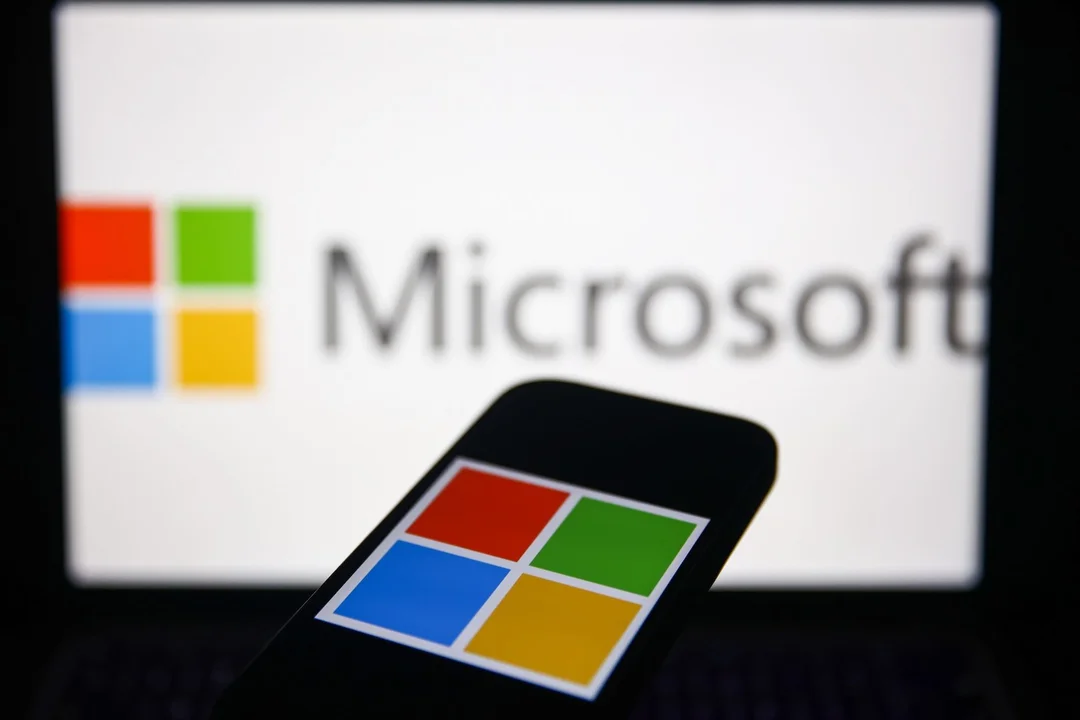
Microsoft’s AI Revolution: Phi Models Redefine Efficiency and Accessibility
Microsoft is making waves in the AI world with its innovative Phi family of models, challenging the status quo of large, resource-intensive systems. The latest additions, Phi 4 mini reasoning, Phi 4 reasoning, and Phi 4 reasoning plus, promise to bring powerful AI capabilities to a wider range of devices and applications. This development is significant because it addresses the growing demand for efficient AI solutions that can operate in low-latency environments without sacrificing performance.
The core of Microsoft's innovation lies in its emphasis on reasoning models. These models are designed to spend more time fact-checking solutions to complex problems, enabling them to excel in tasks that require multi-step decomposition and internal reflection. According to Microsoft, reasoning models are emerging as the backbone of agentic applications with complex, multi-faceted tasks.
Phi 4 mini reasoning, a 3.8 billion parameter model, is specifically designed for educational applications like embedded tutoring on lightweight devices. Trained on roughly 1 million synthetic math problems, it offers a balance of efficiency and advanced reasoning ability. Microsoft's blog post notes: "Using distillation, reinforcement learning, and high-quality data, these [new] models balance size and performance. They are small enough for low-latency environments yet maintain strong reasoning capabilities that rival much bigger models."
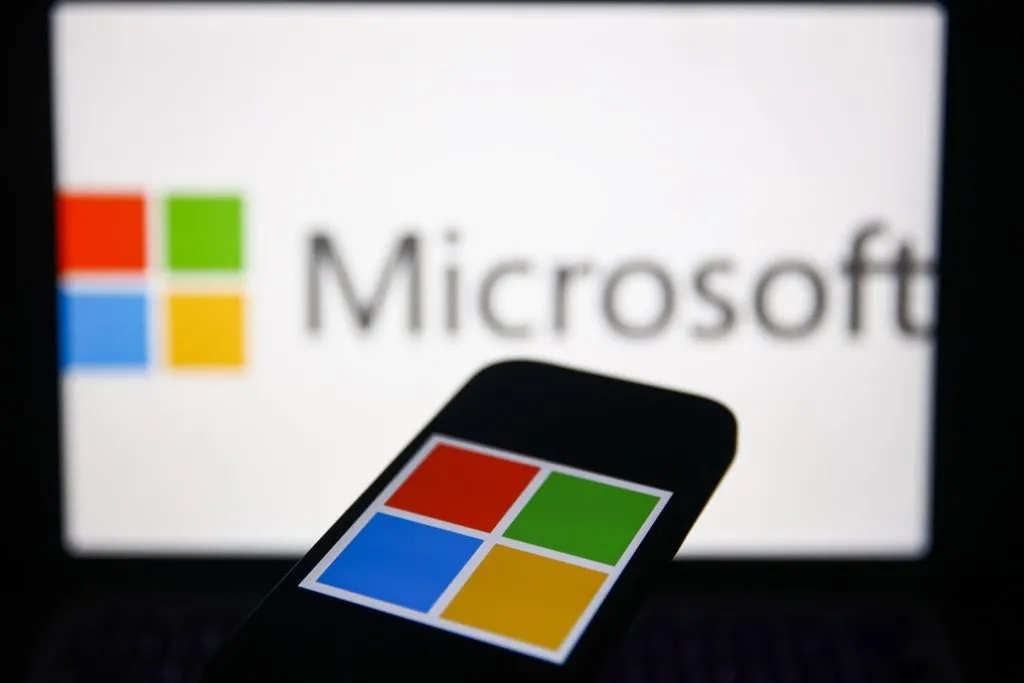
Phi 4 reasoning, a 14-billion-parameter model, stands out due to its training on high-quality web data and curated demonstrations from OpenAI's o3-mini. It demonstrates that smaller models can compete with larger counterparts through meticulous data curation. Even more impressively, Phi 4 reasoning plus, through reinforcement learning, approaches the performance levels of the DeepSeek-R1 model, which has a staggering 671 billion parameters.
These models are not just about size; they are about strategic optimization. The development team at Microsoft leveraged reinforcement learning and high-quality data to balance size and performance, enabling resource-limited devices to perform complex reasoning tasks efficiently. The results are compelling: Phi-4-reasoning and Phi-4-reasoning-plus models outperform the base model Phi-4 by significant margins and show competitive performance against the Deepseek-R1 model across various reasoning and general capabilities.
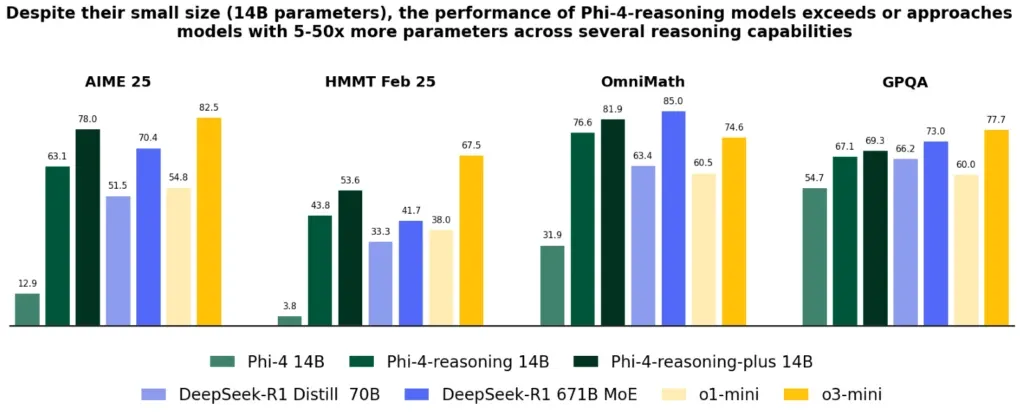
Furthermore, Microsoft is exploring alternative computing architectures to enhance AI efficiency. The introduction of BitNet b1.58 2B4T, an AI model that runs on regular CPUs instead of GPUs, represents a potential paradigm shift. This model stores and processes weights using only three values (-1, 0, 1), allowing for simpler CPU-based processing and significantly reduced energy consumption. If this holds up, users could run chatbots directly on their computers or phones without relying on data centers.
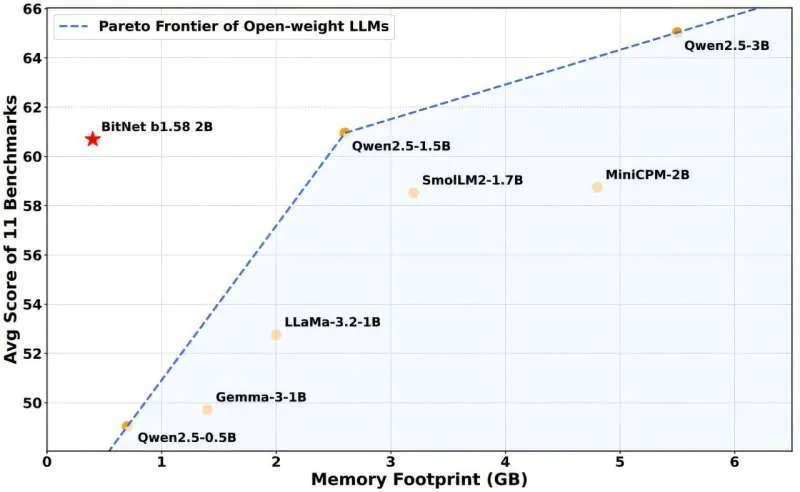
What do these developments mean for the future of AI? Microsoft's focus on efficient, accessible, and versatile AI models signals a move towards democratizing AI technology. The Phi family of models and the BitNet architecture could potentially reduce the energy footprint of AI, enhance user privacy, and open up new possibilities for edge computing and mobile applications.
What are your thoughts on Microsoft's approach to AI? Will smaller, more efficient models become the norm, or will the industry continue to prioritize larger, more powerful systems? Share your opinions in the comments below!