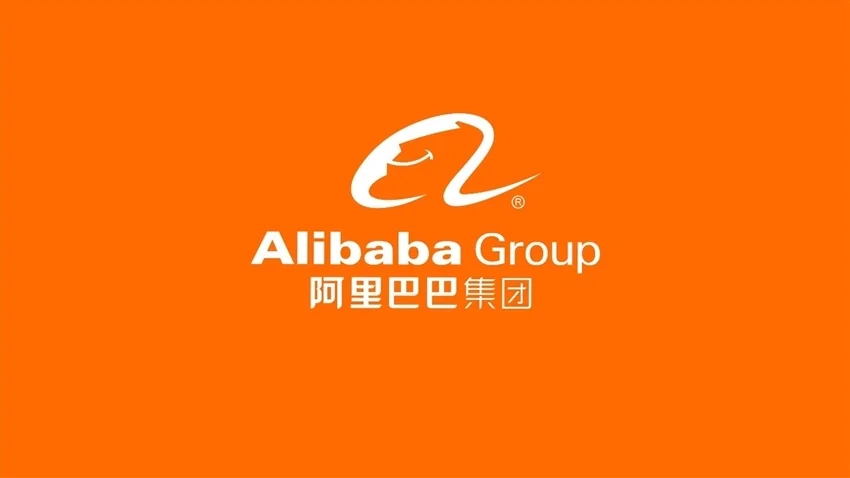
Alibaba’s ZeroSearch: AI Learns to ‘Google’ Itself, Cutting Training Costs by 88%
In a groundbreaking development, Alibaba has unveiled its ZeroSearch technology, a novel approach that empowers AI systems to master information retrieval without relying on expensive, traditional search engine APIs. This innovation slashes training costs by a remarkable 88 percent, potentially revolutionizing the AI development landscape.
The core of ZeroSearch lies in its ability to train large language models (LLMs) to develop advanced search capabilities through a simulation-based approach. Instead of interacting with real search engines during training, the AI learns within a controlled environment, eliminating the need for costly API calls to services like Google Search.
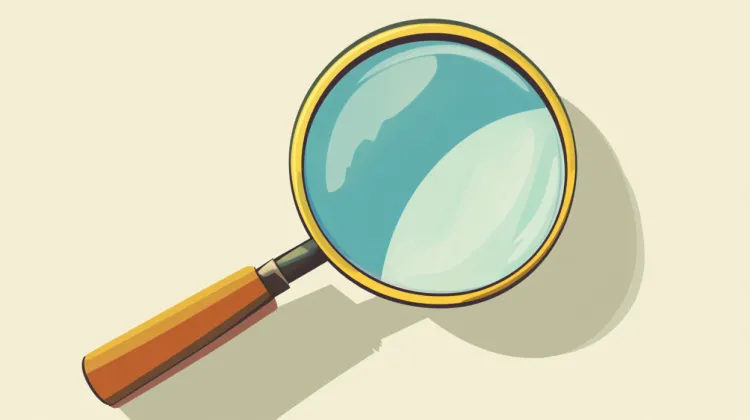
According to the researchers behind ZeroSearch, reinforcement learning (RL) typically requires frequent rollouts, involving hundreds of thousands of search requests, which can lead to substantial API expenses and hinder scalability. ZeroSearch addresses these challenges by incentivizing the search capabilities of LLMs without any interaction with real search engines.
How ZeroSearch Works
Alibaba’s method begins with a supervised fine-tuning process to transform an LLM into a retrieval module. This module can generate both relevant and irrelevant documents in response to a query. During reinforcement learning, the system employs a curriculum-based rollout strategy that progressively degrades the quality of the generated documents. This forces the AI to become more discerning in its search process.
The researchers highlight that LLMs possess extensive world knowledge acquired during large-scale pretraining, enabling them to generate relevant documents given a search query. The main difference between a real search engine and a simulation LLM lies in the textual style of the returned content.
Outperforming Google at a Fraction of the Cost
ZeroSearch has demonstrated impressive results in experiments across seven question-answering datasets. In many cases, it matched or even surpassed the performance of models trained with real search engines. Notably, a 7B-parameter retrieval module achieved performance comparable to Google Search, while a 14B-parameter module outperformed it. The cost savings are significant. Training with approximately 64,000 search queries using Google Search via SerpAPI would cost around $586.70. In contrast, using a 14B-parameter simulation LLM on four A100 GPUs costs only $70.80 – an 88% reduction.
Impact on the Future of AI Development
ZeroSearch represents a major paradigm shift by demonstrating that AI can improve without relying on external tools. This breakthrough has the potential to level the playing field for smaller AI companies and startups with limited budgets, as it drastically reduces the costs associated with training advanced AI systems.
Beyond cost savings, it provides developers with greater control over the training process. With simulated search, developers can precisely control the information the AI sees during training, mitigating the unpredictable quality of results from real-world search engines.
The researchers have open-sourced their code, datasets, and pre-trained models on GitHub and Hugging Face, allowing other researchers and companies to leverage this innovative approach. This move fosters collaboration and accelerates the development of more efficient and cost-effective AI systems.
As LLMs continue to evolve, techniques like ZeroSearch suggest a future where AI systems can develop increasingly sophisticated capabilities through self-simulation, reducing dependencies on large technology platforms. Will this technology reshape the AI landscape and challenge the dominance of traditional search engines? Share your thoughts in the comments below.